Tag: data quality
Data Quality Management Platforms: Ensuring Data Accuracy and Reliability
Written by ew_admin on . Posted in Glossary.
In the digital age, organisations are inundated with vast amounts of information. However, the value of this data is highly dependent on its quality. Poor data quality can lead to incorrect insights, flawed decision-making, and negative business outcomes. Therefore, businesses must employ robust data quality management platforms and tools to ensure the accuracy, reliability, and usability of their information. In this article, we will explore the concept of data quality management and delve into various tools available to organisations for effectively managing information quality.
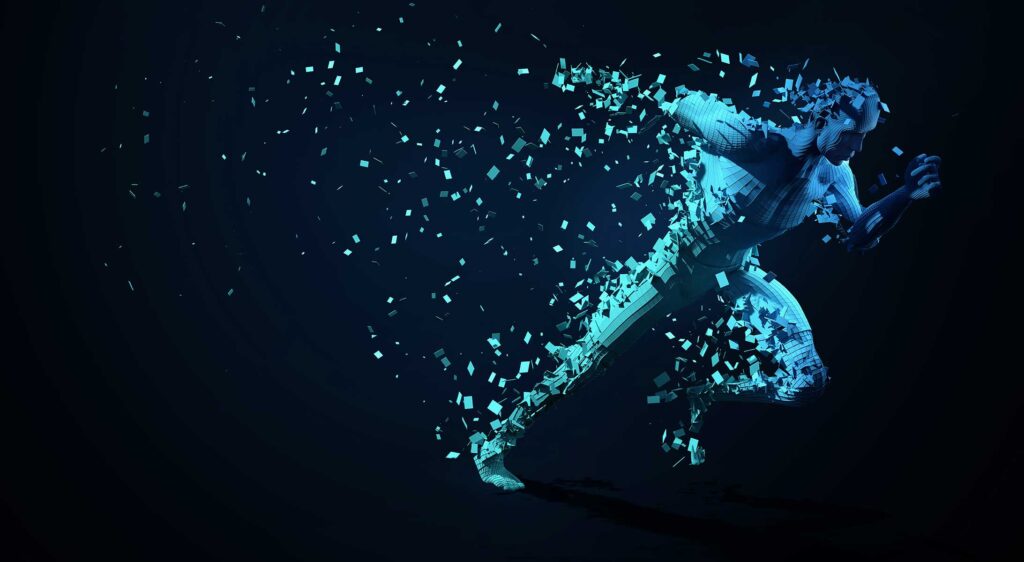
Understanding Data Quality Management
Data quality management refers to the process of ensuring that information meets specific standards and requirements in terms of accuracy, completeness, consistency, validity, and timeliness. It encompasses various activities such as information profiling, information cleansing, information integration, and information governance. It enables organisations to have confidence in their information assets and make informed decisions based on reliable data.
The Need for Data Quality Management Platforms
As information volumes continue to grow exponentially, manual information quality management becomes increasingly challenging and time-consuming. Traditional approaches like spreadsheet-based information cleaning and ad hoc data quality checks are no longer sufficient. Information quality management platforms provide automated, scalable, and comprehensive solutions to address the complexities of managing information quality in modern organisations. These platforms offer a wide range of tools and functionalities that streamline information quality processes, improve efficiency, and enhance overall information governance.
Key Features and Functionalities of Information Quality Management Platforms
Profiling: These tools automatically analyse data sets to identify patterns, anomalies, and inconsistencies. Information profiling enables organisations to gain insights into the structure, content, and quality of their information, helping them understand information-related issues and make informed decisions.
Cleansing: Functionalities that automatically detect and correct data errors, inconsistencies, and inaccuracies. These tools employ various techniques like deduplication, standardisation, validation, and enrichment to cleanse data and ensure accuracy and completeness. This helps organisations eliminate duplicate records, fix formatting issues, and enhance the overall quality of their information.
Data Integration: Enabling seamless data movement across different systems, applications, and databases. These platforms provide robust mechanisms for data mapping, transformation, and synchronisation, ensuring the smooth flow of information between disparate sources. Organisations can leverage information integration capabilities to consolidate data from multiple systems, eliminate data silos, and create a unified view of their information landscape.
Data integration tools support various integration patterns, such as extract, transform, load (ETL), application programming interfaces (APIs), and data virtualisation. These tools enable organisations to extract data from various sources, apply necessary transformations, and load it into a target system or data warehouse. This seamless integration process ensures that information remains consistent and accurate throughout its journey across different systems.
Moreover, information integration functionalities within information quality management platforms often include data validation checks during the integration process. These checks verify the integrity and quality of data being integrated, ensuring that only reliable and accurate data is transferred. By incorporating information quality checks during integration, organisations can identify and address information discrepancies or errors before the data is consumed by downstream applications or processes.
Information integration capabilities also allow organisations to synchronise data between systems in real-time or batch mode. This synchronisation ensures that data across different systems remains up to date and consistent, eliminating information inconsistencies and enabling timely decision-making.
Furthermore, this supports information lineage and metadata management. These platforms capture and document the flow of data across various systems, providing visibility into the information’s origin, transformations, and destinations. This data lineage information helps organisations understand the lifecycle, trace issues back to their sources, and ensure compliance with governance regulations.
Data Standardisation: Information quality management platforms offer information standardisation capabilities that ensure consistency in information formats, values, and structures. These tools help organisations enforce standard information conventions, eliminate information discrepancies, and improve information integrity. Information standardisation ensures that information from various sources is transformed into a unified format, making it easier to analyse and interpret.
Validation: These tools perform data validation checks to ensure that data meets specified criteria, such as data type, range, and referential integrity. Information validation helps identify and rectify information anomalies, reducing the risk of erroneous data being used for decision-making.
Governance: Information quality management platforms often incorporate information governance capabilities that establish policies, rules, and workflows for managing information quality. These tools enable organisations to define information quality standards, assign responsibilities, and track information quality issues throughout the data lifecycle. Information governance ensures that information quality management is an ongoing process and fosters a culture of accountability and transparency.
Monitoring and Reporting: Information quality management platforms provide monitoring and reporting features that enable organisations to track the performance and health of their data. These tools offer real-time information monitoring, data quality dashboards, and comprehensive reports that highlight information quality metrics, trends, and issues. Information monitoring and reporting help organisations proactively identify and address information quality issues, ensuring continuous improvement.
Popular Information Quality Data Management Platforms and Tools
Informatica Data Quality: Informatica Data Quality is a comprehensive information quality management platform that offers a wide range of tools and functionalities. It provides information profiling, information cleansing, information integration, and information governance capabilities. Informatica Data Quality is known for its advanced information profiling capabilities, which enable in-depth analysis of information quality issues.
Talend Data Quality: Talend Data Quality is a powerful information quality management platform that offers information profiling, information cleansing, and information integration features. It provides a user-friendly interface and supports both batch and real-time data processing. Talend Data Quality also offers collaborative features, allowing teams to work together on information quality initiatives.
IBM InfoSphere Information Server: IBM InfoSphere Information Server is an enterprise-grade information quality management platform that provides comprehensive data integration and information quality functionalities. It offers information profiling, information cleansing, and information standardisation capabilities. IBM InfoSphere Information Server also includes robust information governance features, allowing organisations to establish and enforce information quality policies.
SAS Data Management: A feature-rich data quality management platform that offers a wide range of information quality tools and functionalities. It provides information profiling, information cleansing, and information integration capabilities. SAS Data Management also includes advanced analytics capabilities, allowing organisations to gain deeper insights into their information quality.
Oracle Data Quality: A scalable and reliable data quality management platform that offers information profiling, information cleansing, and information integration features. It provides a comprehensive set of information quality tools that can be integrated with Oracle’s broader data management ecosystem. Oracle Data Quality also includes data matching and data enrichment capabilities.
Best Practices for Implementing Data Quality Management Platforms
Clearly define information quality requirements and metrics: Before implementing an information quality management platform, organisations should clearly define their information quality requirements and establish measurable metrics. This ensures that the platform aligns with the specific needs of the organisation.
Establish a framework: Implementing a platform should be accompanied by a governance framework. This framework should define roles, responsibilities, and processes for information quality management, ensuring that information quality is a collective effort across the organisation.
Invest in data profiling and analysis: Profiling and analysis play a crucial role in understanding the current state of information quality. Organisations should invest time and resources in thorough information profiling to identify information anomalies, patterns, and inconsistencies. This analysis helps in prioritising information quality issues and developing appropriate strategies for information cleansing and improvement.
Implement checks at multiple stages: Businesses should implement information quality checks at various stages of the data lifecycle. This includes entry, integration, migration, and transformation processes. By incorporating checks at multiple touchpoints, organisations can identify and rectify any issues early on, minimising the impact on downstream processes.
Establish information quality monitoring and reporting mechanisms: Implementing an information quality management platform should include setting up robust information quality monitoring and reporting mechanisms. Organisations should regularly monitor information quality metrics, track trends, and generate comprehensive reports to identify areas of improvement and measure the effectiveness of information quality initiatives.
Provide adequate training and support: To ensure successful adoption and utilisation of information quality management platforms, organisations should provide proper training and support to their teams. Users should be familiarised with the functionalities and features of the platform and trained on best practices for information quality management. Ongoing support and guidance should be provided to address any queries or challenges that arise during the implementation process.
Foster a data-driven culture: Successful information management relies on fostering a data-driven culture within the business. This involves promoting the importance of information quality, encouraging data ownership and accountability, and emphasising the value of accurate and reliable information for decision-making.
In an era where data is hailed as the new currency, ensuring information accuracy, reliability, and usability is of paramount importance. Data quality management platforms offer a wide range of tools and functionalities that enable organisations to effectively manage information quality and mitigate the risks associated with poor quality. By employing these platforms and following best practices for implementation, organisations can unlock the true potential of their information assets and make informed decisions based on reliable data. Investing in information quality management platforms is an investment in the long-term success and competitiveness of businesses in today’s data-driven landscape.
Contact us to talk about how we can help you get your business data environment ready to scale up.
Data governance
Written by ew_admin on . Posted in Glossary. No Comments on Data governance
It involves establishing policies, standards, and procedures for the management of data assets, including how data is collected, stored, processed, shared, and used across different systems and departments.
The goal of data governance is to provide a framework for managing data assets effectively, reducing the risk of data loss, improving data quality, and ensuring compliance with regulatory requirements.
A company, for example, could use data governance to save money in several ways:
- Improve data quality: By implementing data governance policies and procedures that ensure the accuracy and completeness of data, a company could reduce the number of errors and inconsistencies in their data. This could help the company identify billing errors or other issues that might be causing them to lose money.
- Identify inefficiencies: By having a comprehensive view of their data, a company could identify areas where they might be overspending or wasting resources. For example, by analyzing data on energy usage, the company could identify buildings or facilities that are using more energy than they should be and take steps to reduce consumption.
- Streamline operations: By having a centralised view of their data, a company could identify areas where they might be able to streamline operations and reduce costs. For example, by analyzing data on equipment maintenance, the company could identify areas where they might be able to reduce downtime and maintenance costs.
- Ensure compliance: Companies are often subject to regulations and reporting requirements that require them to maintain accurate and complete data. By implementing a data governance program that ensures compliance with these requirements, a company could avoid fines or penalties that could cost them money.
Data compliance refers to the practice of adhering to established standards and regulations concerning the collection, storage, processing, and sharing of data.
Data compliance is essential to ensure that personal data is being handled in a responsible and ethical manner, while also protecting the rights of individuals and avoiding legal consequences.
Data compliance standards can vary depending on the industry and the type of data being handled. For example, healthcare organizations must comply with HIPAA regulations to protect patient information, while financial institutions must comply with GDPR or CCPA regulations to protect customer financial information.
Organizations that fail to comply with data regulations face legal penalties, which can include fines, lawsuits, and even criminal charges in some cases. As a result, ensuring data compliance is crucial for any organization that handles personal or sensitive information.
A company should invest in being compliant with their data management for several reasons:
- Legal Compliance: Non-compliance with data management regulations can result in heavy fines, lawsuits, and legal penalties, which can have a severe impact on a company’s reputation and financial health.
- Protects customer trust: Compliance with data management regulations helps build customer trust by demonstrating the company’s commitment to protecting their personal data. Customers are more likely to trust a company that takes data management seriously.
- Competitive Advantage: Compliance with data management regulations can give a company a competitive advantage over its competitors, as it ensures a higher level of data security and privacy.
- Risk Management: Data breaches can result in significant financial loss and reputational damage. Compliance with data management regulations helps mitigate this risk by implementing best practices and security measures to prevent data breaches.
- Good Corporate Citizenship: Complying with data management regulations is a way for companies to be good corporate citizens by acting responsibly and ethically in the handling of personal data. This can enhance the company’s reputation and improve customer loyalty.
Overall, data governance can help a company make more informed decisions, reduce risk, and improve operational efficiency, all of which can help save money in the long run.
Data Quality
Written by ew_admin on . Posted in Data services.
Data quality
Written by ew_admin on . Posted in Glossary. No Comments on Data quality
Data quality is the degree to which data is fit for its intended purpose in terms of its usability and reliability. High-quality data is essential for effective decision-making, analysis, and reporting.
Accurate data is free from errors and is a true reflection of reality. Completeness refers to having all the necessary data elements for a specific purpose or analysis. Consistency ensures that data is standardised and uniform across all sources. Timeliness ensures that data is available when needed and is up-to-date. Finally, relevance ensures that the data is useful and applicable to the specific task or analysis at hand.
Poor data quality can lead to inaccurate analysis, flawed decision-making, and miscommunication. It can also lead to wasted time and resources spent on correcting errors and inconsistencies. As a result, ensuring high-quality data is essential for any organisation that relies on data to make informed decisions and drive business outcomes.
There are several ways to improve data quality:
- Data Governance: Establishing a robust data governance framework can help ensure that data is accurately collected, processed, and stored.
- Data Profiling: Conducting data profiling helps identify data quality issues by analyzing data characteristics such as completeness, accuracy, and consistency.
- Data Standardisation: Standardizing data by using consistent naming conventions, data formats, and data definitions can help ensure that data is consistent and uniform across all sources.
- Data Cleansing: Data cleansing involves identifying and correcting errors, inconsistencies, and inaccuracies in data.
- Data Validation: Data validation involves checking data accuracy and completeness by comparing it to known values, rules, and criteria.
- Data Verification: Data verification involves checking data accuracy and completeness by comparing it to external sources or other data sets.
- Data Quality Metrics: Establishing data quality metrics helps measure the effectiveness of data quality initiatives and identify areas for improvement.
Improving data quality requires a combination of technical and organisational efforts. By establishing a robust data governance framework, conducting data profiling, standardiSing data, cleansing data, validating data, and measuring data quality metrics, organisations can improve their data quality and ensure that data is reliable and fit for its intended purpose.