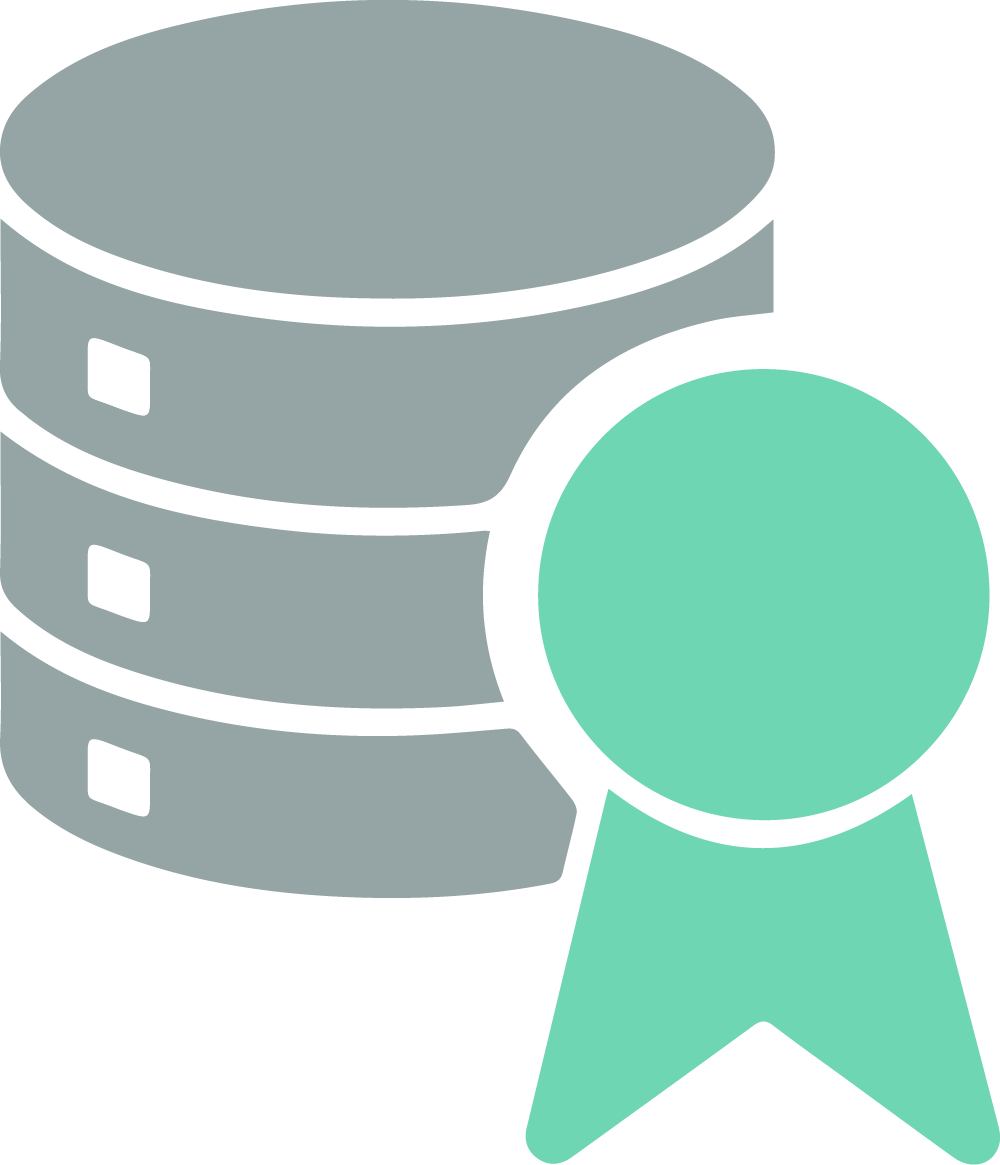
Data quality
Data quality is the degree to which data is fit for its intended purpose in terms of its usability and reliability. High-quality data is essential for effective decision-making, analysis, and reporting.
Accurate data is free from errors and is a true reflection of reality. Completeness refers to having all the necessary data elements for a specific purpose or analysis. Consistency ensures that data is standardised and uniform across all sources. Timeliness ensures that data is available when needed and is up-to-date. Finally, relevance ensures that the data is useful and applicable to the specific task or analysis at hand.
Poor data quality can lead to inaccurate analysis, flawed decision-making, and miscommunication. It can also lead to wasted time and resources spent on correcting errors and inconsistencies. As a result, ensuring high-quality data is essential for any organisation that relies on data to make informed decisions and drive business outcomes.
There are several ways to improve data quality:
- Data Governance: Establishing a robust data governance framework can help ensure that data is accurately collected, processed, and stored.
- Data Profiling: Conducting data profiling helps identify data quality issues by analyzing data characteristics such as completeness, accuracy, and consistency.
- Data Standardisation: Standardizing data by using consistent naming conventions, data formats, and data definitions can help ensure that data is consistent and uniform across all sources.
- Data Cleansing: Data cleansing involves identifying and correcting errors, inconsistencies, and inaccuracies in data.
- Data Validation: Data validation involves checking data accuracy and completeness by comparing it to known values, rules, and criteria.
- Data Verification: Data verification involves checking data accuracy and completeness by comparing it to external sources or other data sets.
- Data Quality Metrics: Establishing data quality metrics helps measure the effectiveness of data quality initiatives and identify areas for improvement.
Improving data quality requires a combination of technical and organisational efforts. By establishing a robust data governance framework, conducting data profiling, standardiSing data, cleansing data, validating data, and measuring data quality metrics, organisations can improve their data quality and ensure that data is reliable and fit for its intended purpose.